Would you consent to a surveillance system that watches without video and listens without sound?
If your knee-jerk reaction is “no!”, then “huh?” I’m with you. In a new paper in Applied Physics Letters, a Chinese team is wading into the complicated balance between privacy and safety with computers that can echolocate. By training AI to sift through signals from arrays of acoustic sensors, the system can gradually learn to parse your movements—standing, sitting, falling—using only ultrasonic sound.
To study author Dr. Xinhua Guo at the Wuhan University of Technology, the system may be more palatable to privacy advocates than security cameras. Because it relies on ultrasonic waves—the type that bats use to navigate dark spaces—it doesn’t capture video or audio. It’ll track your body position, but not you per se.
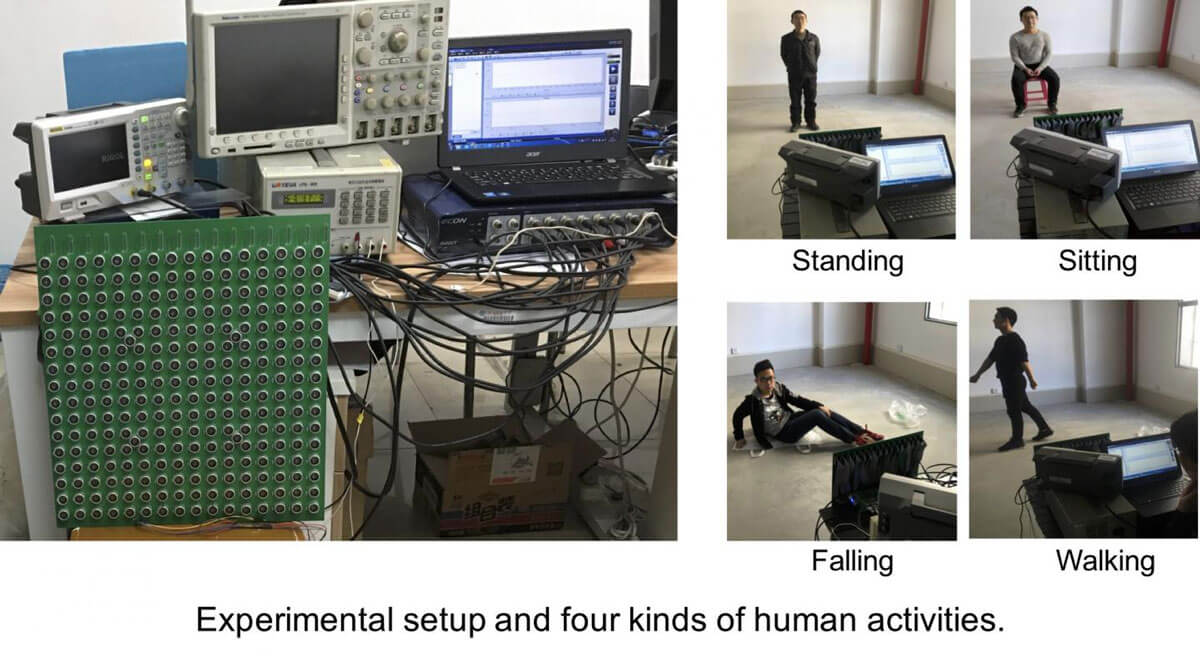
When further miniaturized, the system could allow caretakers to monitor elderly folks who live alone for falls inside their house, or track patient safety inside hospital rooms. It could even be installed in public areas—trains, Ubers, libraries, park bathrooms—to guard against violence or sexual harassment, or replace video cameras in AirBnB homes to protect both the property and the guest’s privacy.
Because the system only detects body movement, there’s no issue with facial recognition—or perhaps any identification—based on recordings alone. The system doesn’t even generate blob-like body shapes that grace US airports’ body camera screens. It’s monitoring, yes, but with a thin veil of privacy, similar to leaving semi-anonymous comments online.
At least that’s the pitch. If you’re a tad skeptical, so am I. San Francisco recently banned facial recognition technology, and New York may soon follow with even more stringent surveillance rules. But in countries where security cameras range in the hundreds of thousands and privacy isn’t necessarily a basic right, an echolocating monitoring system may better appease folks who are increasingly uncomfortable having their every move watched and recorded.
“Protecting privacy from the intrusion of surveillance cameras has become a global concern. We hope that this technology can help reduce the use of cameras in the future,” said Guo.
How Does It Work?
The team took a hint from bats and other animals that use echolocation as their main navigational tool.
To echolocate, you need two main types of hardware: a sensor, such as a microphone, that blasts out ultrasonic waves to bounce off surfaces, and a receiver that collects the reflected waves. According to Guo, previous attempts at machine echolocation often only used a single microphone and a handful of sensors. Effective, yes, but not efficient, like a somewhat handicapped bat.
“The recognition accuracy was not very high” at roughly 90 percent, the authors said. For a system to work as well as video cameras, the accuracy needs to be near perfect.
The team set up four emitters in a three-dimensional space, with each shooting out sound waves at 40kHz—roughly two times above the highest hearing ability of a healthy young person. To capture the rebounding waves, they used a 256 acoustic array, perfectly lined up on a single surface in a 16 by 16 grid. Both transmitters and receivers are physically located on a single chip-like structure, visually similar to round seeds dotting a green lotus pod.
Each time a volunteer moves in front of the array—standing, sitting, falling, or walking—the receiver scans through the blanket of reflected sound waves, one row at a time. In all, the team had four people of different heights and weights come in, allowing the system to better generalize a particular data pattern to a movement rather than to a certain person.
Now comes the brainy part. To mimic bat-brain processing in computers, the team turned to a convolutional neural network (CNN), the golden child and driving horse of many current computer vision systems. They designed an algorithm that first pre-processes all the echolocation data to strip out noise—anything the sensors picked up outside the target 40kHz, give or take 5kHz for leniency.
The algorithm then parsed the gathered data over time to fish out movement patterns, similar to how brain-machine interfaces find muscle intent in neural electrical signals. For example, sitting reflects a slightly different pattern of sound waves than standing or falling. Similar to other deep neural networks, it’s impossible to explain how each body position differs in terms of echolocation, but the acoustic fingerprints are distinct enough for the algorithm to successfully parse out the four tested activities 97.5 percent of the time.
In general, the algorithm seems to better identify static activity such as sitting and standing rather than movements. This is expected, explained the authors, because falling and walking introduce individual differences in how people move, making it harder for computers to figure out a general acoustic pattern.
Big Brother?
Guo’s study further expands a relatively new field called human activity recognition. Here, computers try to predict the movement of a person based on sensor data alone. It might sound incredibly “big brother,” but anyone who has a FitBit, Apple Watch, or other activity trackers has already reaped the benefits—human activity recognition is how your smartwatch counts your steps using the embedded gyroscope. The field also encompasses video surveillance, such as computers figuring out what a person is doing based on pixels in images or videos. Have a Microsoft Kinect? That nifty box uses infrared light, a video camera, and depth sensors to identify your movement while gaming.
“Human activity recognition is widely used in many fields, such as the monitoring of smart homes, fire detecting and rescuing, hospital patient management, etc,” the authors explained.
As sensors increasingly become lightweight the technology will only expand. In 2017, a Chinese-American collaboration found that it’s possible to track human movements based on surrounding WiFi alone. Many of these systems are still too large to be completely portable, but hardware miniaturization is almost inevitably in the future.
Not everyone protests against increased monitoring. Caregivers in particular might appreciate the technology to alert them to the elderly falling—something innocuous when young but deadly after a certain age. The authors envision a fully automated system in which a fall automatically alerts multiple sources of help, without necessarily leaking what the person is doing before the fall.
But good intentions aside, Guo’s system screams of misuse potential. In stark contrast to facial recognition, little discussion has so far focused on privacy issues surrounding human activity tracking. According to Jake Metcalf, a technological ethicist at the think tank Data & Society in New York, the system could easily be repurposed to listen in on peoples’ private lives, or combined with existing technologies to further increase surveillance coverage.
For now, Guo’s team is reluctant to weigh in on the privacy ramifications. Rather, his team hopes to further tailor the system to more complex activities and “random” situations where a person may be lounging about.
“As we know, human activities are complicated, taking falling as an example, and can present in various postures. We are hoping to collect more datasets of falling activity to reach higher accuracy,” he said.
Image Credit: ioat / Shutterstock.com